How Big Data Analytics Impacts Manufacturing & Supply Chain
Since the start of COVID-19, there has been a global disruption to the supply chain of manufacturing companies. The situation is worse for companies operating within the EU, mainly due to political unrest and tensions in Central Europe. Raw material shortages, rising energy costs, transportation disruptions, and inflation are issues affecting the manufacturing industry in the region. Economic losses resulting from these supply chain disruptions in Europe are estimated to be as high as €112.7 billion and could rise to €242 billion this year.
Consequently, the affected organizations must adjust by adopting technologies and practices that mitigate the threats to efficient supply chain management and provide the best conditions for profitability. Enter big data analytics, the complex but reliable solution unlocking new possibilities for optimizing supply chains in manufacturing.
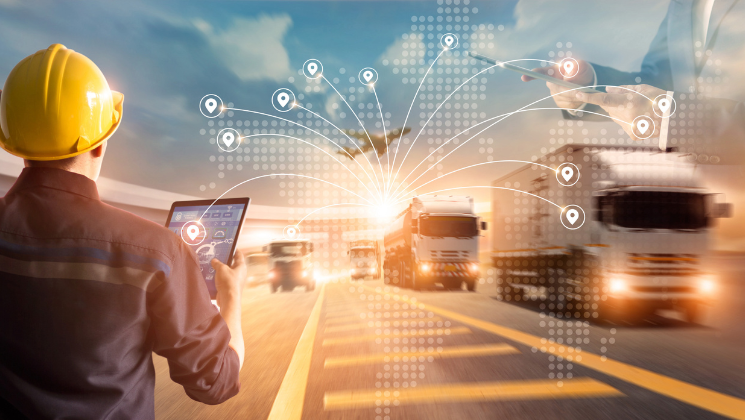
Big data analytics for supply chain optimization
Big data analytics uses advanced analytic techniques and algorithms to reveal hidden patterns and extract actionable insights from large and diverse data sets. Characterized by high volume and a mix of structured, semi-structured, and unstructured data from various sources, big data exceeds the capabilities of traditional processing systems. Hence, advanced analytic models are necessary for fast and efficient analysis. By leveraging big data analytics, businesses can make data-driven decisions and enhance operational efficiency.
Supply chain management is the comprehensive set of practices and processes involved in the production, sales, and distribution of products to customers. It begins with acquiring and transforming raw materials into finished products ready for sale. It extends to logistics management which involves moving these products from the warehouses to the destinations of the final consumers. Because these processes are critical to the organization's success, every manufacturing company’s goal is to ensure a high-performing and optimized supply chain.
Manufacturing companies will inevitably encounter large data in the supply chain optimization process. This is due to the increased use of artificial intelligence (AI), the Internet of Things (IoT), and mobile devices, all of which have a high volume of data and multiple structures. Big data can originate from various sources like sensor outputs, high-resolution videos, and many more which can easily reach orders of terabytes or petabytes. Audio files, log files, website & social media activity can also amount to large data over a prolonged time of monitoring production, logistics, and sales.
Benefits of big data analytics in supply chain optimization
Big data analytics has undoubtedly become an invaluable asset in supply chain management. The insights arising from advanced analytics have allowed businesses to identify inefficiencies, respond to changing market trends, and implement data-driven solutions to optimize production and ensure customer satisfaction. The benefits of data analytics in supply chain optimization include the following:
- Improved demand forecasting.
Big data analytics empowers companies to use historical demand data and current market trends to predict demand accurately. Subsequently, businesses can adjust their production schedules to meet customer demands.
- Real-time tracking.
Businesses can accurately track inventory levels and other aspects of the supply chain by analyzing data from sensors and other sources. This allows for better inventory level management and supply chain visibility which curb wastage and reduced lead time.
- Predictive maintenance of equipment.
Because equipment downtime puts the supply chain at risk, manufacturers must prioritize equipment health and performance. By gathering and analyzing sensor and historical maintenance data, businesses can accurately predict equipment malfunction and institute pre-emptive corrective actions.
- Enhanced Logistics Management.
Logistics management involves minimizing transportation costs and optimizing delivery times, and it impacts customer experience and revenue generation. Employing the robust capabilities of big data analytics can help manufacturers plan important logistics better.
- Smoother Supplier Relationship Management.
Analyzing supplier data helps businesses objectively assess supplier performance and quality. This informs decisions on the best suppliers to work with, protecting the integrity of the supply chain and improving product quality as a result.
Common challenges in big data analytics for supply chain management
Implementing big data analytics comes with a few challenges. The most significant one is data quality. The quality of data impacts the accuracy of insights arising from big data analytics. Therefore, businesses must eliminate incomplete and inaccurate data to prevent poor decision-making. Integrating data from various sources is another challenge. Failure to optimize data integration often leads to information silos which affect the reliability of insights.
Additionally, businesses could be vulnerable to data breaches if privacy and security measures are not enforced while handling the substantial amounts of sensitive data in their system. The availability of individuals with the specialized skills and expertise to manage advanced analytics systems may also hinder the handling of big data.
Overall, these challenges can significantly affect the application of big data analytics in supply chain optimization. Hence, businesses must proactively find suitable solutions to these problems at the implementation stage to maximize the benefits of advanced analytics.
Key steps for implementing big data analytics in supply chain optimization
A strategic approach to implementing big data analytics improves the chances of successfully optimizing the supply chain network. Here are five important steps to follow:
1. Define objectives and identify relevant data sources
Start your implementation process by identifying your supply chain's unique problems and challenges. By doing so, your company will be able to outline goals and objectives relevant to your business model. Subsequently, identify all the relevant data sources needed for efficient big data analytics, and ensure accuracy and completeness. If necessary, synthetic data can be generated to replace missing data so that machine learning models are professionally trained.
2. Develop data models
The next step for implementing big data analytics is to develop or adopt models for processing data and reporting insights. Be sure to select the data models that best fit the goals and objectives outlined earlier.
3. Implement necessary infrastructure and tools
Big data analytics only thrives in an ecosystem of hardware, software, and network infrastructure for storing and processing large volumes of data. Your brand must identify the infrastructure and tools necessary to realize your long-term plans, such as cloud-based solutions and data visualization software.
4. Train personnel and establish processes
Your team's skill level significantly affects your implementation program's success. So, regular training of data scientists, analysts, and other personnel involved in the supply chain is essential. Additionally, key processes such as data security protocols and governance policies must be established early in the project.
5. Monitor and continuously improve performance
After launching your big data analytics program, measure performance against defined goals and objectives. Continuously improve your system by introducing new data sources and updating data models to maintain a competitive edge over other manufacturers.
Real-world examples of big data analytics in manufacturing supply chain
Numerous manufacturers across the globe are beginning to adopt big data analytics for their supply chain management. There is a good number of successful case studies to urge more companies to follow this trend. A strong example is that of global manufacturer Lenovo, which uses IBM Sterling Supply Chain Insights with Watson, an AI-powered third-party software solution, to deliver cognitive and predictive analytics for its supply chain management process. This has helped Lenovo ensure visibility across the entire supply chain while drastically reducing its response time to disruptions.
Siemens, another manufacturing giant, uses big data analytics in its train production division. It collects data from train sensors and analyses it for patterns and trends predictive of failure so repairs can be conducted appropriately. This ensures that companies that Siemens supplies trains enjoy more mileage with fewer trains and rarely experience disruption in their transportation services. For context, Renfe, a Spanish train operator using Siemens' products, only experience one five-minute delay in 2,300 journeys, demonstrating the efficiency of the German manufacturer's predictive maintenance program.
Developing trends in big data analytics for supply chain management to watch
Emerging technologies in big data analytics, including artificial intelligence, blockchain technology, the Internet of Things (IoT), and cloud computing, have the potential to take supply chain optimization to unprecedented levels of efficiency and performance. Artificial intelligence and machine learning will provide more advanced analytical power, empowering businesses to perform more accurate forecast demand and inventory monitoring. Blockchain technology can help manufacturing companies improve transparency, traceability, and security of data exchange.
Internet of Things (IoT) improves connectivity between hardware and software through sensors that gather and disseminate real-time data. Finally, cloud computing will solve the issue of increasing data volumes by providing unlimited storage and impressive computational power. Adopting these recent technologies will undoubtedly enhance the reliability of data analytics and decision-making, ensuring optimal supply chain performance.
The Power of Data Analytics in Supply Chain
Every manufacturing company aims to create rich customer experiences and record high-profit margins. The operational efficiency of the supply chain is critical to achieving those goals. With big data analytics helping businesses improve their demand forecasting, inventory monitoring, predictive maintenance, and logistics management, the power to optimize supply chains is universally accessible to companies, provided a strategic and stepwise approach is taken in implementing this technology.
ACTUM Digital can help your business leverage the power of big data analytics for supply chain optimization. Find out how our advanced system integration can help your organization overcome common challenges and meet specified objectives here.
You may also like...
[04/05/2023] Optimizing Production Efficiency with Predictive Maintenance
In the rapidly evolving world of manufacturing, efficiency remains a constant watchword. Businesses are continuously searching for new methods and strategies to boost efficiency...
Read the Insight